

A system that does not use the POS of the to- kens is the one of Reynar and Ratnaparkhi (1997), based on a Maximum Entropy model. Mikheev (2000) reported a 0.25% error rate on the Brown corpus and a 0.39% error rate on WSJ with a system that uses the POS tags of the po- tential token and the two POS of the previous tokens. An accuracy of 99.7% was established for sentences that end in period. Grefenstette and Tapanainen (1994) built a system that uses regular expressions and a list of the most frequent abbreviations. Their system uses the context to determine a potential sentence mark (the POS tags of six words before the potential mark and six words after it). They report 98.5% and 98.9% accuracy on the Wall Street Journal (WSJ).
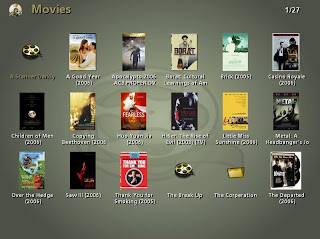
One of the best systems that perform the task of sentence detection is Palmer and Hearst’s (1997) system. The performance of any of these applications can be improved if an accurate SBD system is used. Some of the files have the charac- ters with Romanian diacritics, while some do not.ĭetermining the sentence boundaries in a text is an important task for many Natural Language Processing systems – machine translation, pars- ing, information extraction, summarization, etc.
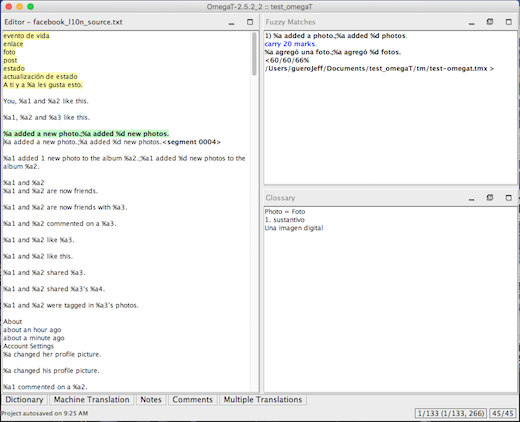
The training corpus for the Romanian Lan- guage contains texts from different fields (litera- ture, history, science, medicine, etc.) collected from the Web. Also, special characters might not be used in a given text, that is, the texts are written without the diacritics for the special characters. What if there is a quote in an English sentence that uses only one word from the Romanian language that has a special character? This problem can appear in any other language that has specific charac- ters. Even though there are special diacritics in the alphabet of the Romanian language, we cannot rely only on them for Language Identification. Romanian is in a way similar to Span- ish, Italian, and French, and a language identifi- cation process on a short text can be easily “fooled” if not using the right features. Influence, Romanian is not a very easy language to learn. The formula that the Naïve Bayes classifier uses to determine the probability of a new text being in one of the languages supported by the system is: It will also provide the probability values for all languages. BALIE guesses the language of a tested text as being the lan- guage that has the highest probability. The language identification module of our sys- tem is based on the work of Beesley (1999). For now, we used bigrams and unigram frequencies. The learning process is done using n-grams (sequences of n charac- ters). For each language, we used a corpus (approximately 50 files, several pages long) for training and around 28 files for testing. BALIE deals with this task from a machine learning point of view, creating a Language Model for each of the supported languages. No inform ation about the size of the documents is provided. They reported a 98% accuracy using a cosine vector comparison. Takei and Sogukpainar (2004) built a system that uses unigram frequencies for classifying 4 languages. The method that they used is not mentioned and neither is the size of the training files. The system will also give as a result the name of the languages that are most similar with the one that it was guessed. System supports 260 languages in different character encodings, with an accuracy of almost 100% for texts of minimum 250 characters.
